Intelligent automation – a blend of robotic process automation and artificial intelligence solutions – can deliver huge efficiency gains, fast.
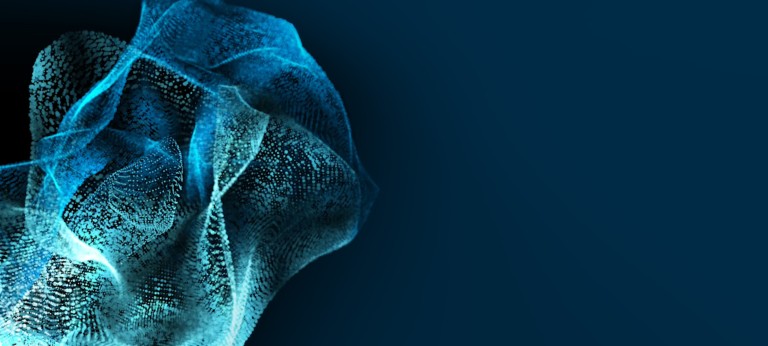
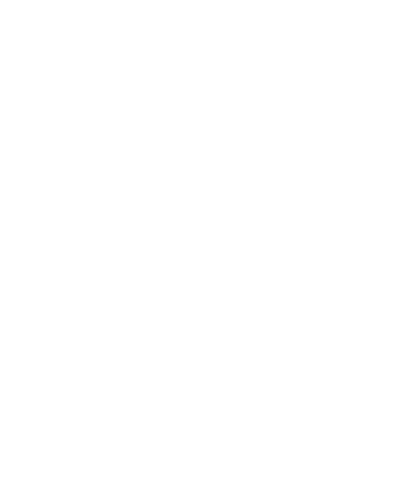
AI-driven commodity price optimization
By Oliver Knapp and Pieter Niehues
CostIQ – Roland Berger’s approach to data-driven, AI-powered raw material procurement
In this article, we take a closer look at how commodity purchasing firms can optimize prices in uncertain, volatile markets. We focus on the importance of artificial intelligence and advanced analytics, and explain how AI-driven commodity price optimization can yield cost savings of up to 5%.
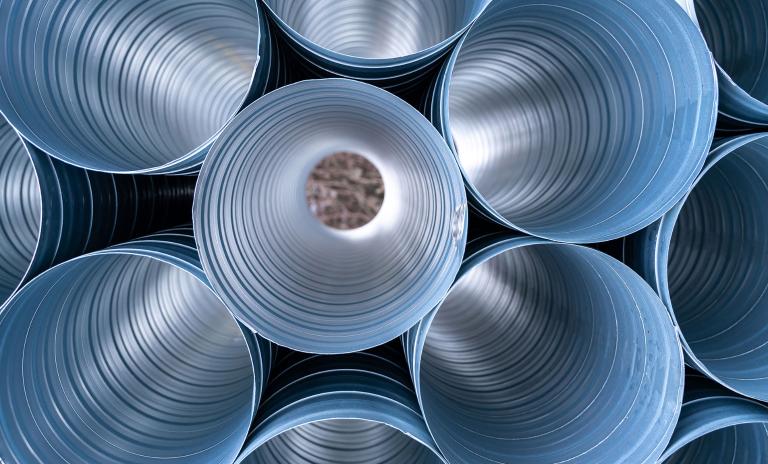
Since January 2020, prices across all major commodity classes have risen significantly, posing a serious challenge to commodity processing firms. Aluminum prices, for instance, had almost doubled by early 2022. It’s a similar story for other industrial metals as well as for energy, chemicals, agricultural products and ocean shipping rates.
Many commodity prices may have stabilized in recent months, but they are still up to 45% higher than 2020 levels. And according to the latest futures price data from major commodity exchanges such as the London Metal Exchange (LME) or the New York Mercantile Exchange (NYMEX), they are unlikely to fall significantly over the next six months. For companies with commodity-intense products, commodity prices will continue to pose a high planning risk as well as add significant costs and margin pressure.
Four ways to optimize commodity purchasing
So, how can commodity purchasing companies overcome these challenges to reduce costs, increase profit margins and minimize risk?
Roland Berger’s commodity price optimization approach contains four main elements:
- Cost optimization towards the supplier
- Margin optimization incorporating the customer
- Risk minimization leveraging the financial market
- Working capital optimization through advanced inventory planning
"With our AI-based CostIQ approach, we continuously help companies identify significant opportunities for cost and risk reduction and optimize purchasing decisions considering market developments. This enables our clients to save up to 5% of raw material procurement costs even in a volatile market environment."
Cost optimization focuses on optimizing price agreements with raw material suppliers via fixed-price contracts for a specific period, index-based price formulas or effective price renegotiations. This also includes claim defense and cost clawbacks if suppliers raise their prices disproportionately to rising raw material and energy costs or fail to pass on falling market prices.
Margin optimization aims at passing on rising purchase prices to the customer. This requires appropriately designed price adjustment clauses in contractual agreements with the customer. The approach can be particularly effective if your own market power is high and/or the market power of the customer is low.
Risk minimization looks to externalize risk towards the financial market. This can be done by optimizing forward contracting at exchanges like the LME for industrial metals, the Baltic Exchange for freight, the European Energy Exchange (EEX) for energy or the Chicago Board of Trade (CBOT) for agricultural products, where monthly or quarterly contracts are traded for future delivery at a known price. This reduces price risk compared to the spot market, where price movements are very hard to predict. Futures prices can also indicate expected market movements.
Working capital optimization aims at optimizing inventory levels while considering price fluctuations. This may involve buying in advance whenever there is an attractive price opportunity, for instance, or consuming directly from storage when prices are rising. The benefit of forward buying must also be weighed up with inventory holding cost and the cost of capital, which have become increasingly relevant since 2022.
Each of these four elements comes with specific challenges for procurement managers: When is the right time to buy? What is the right quantity to buy in advance and store? What is the correct mix of instruments considering spot and forward or futures markets? Or what is the right index formula for a price adjustment clause in a contract with suppliers or customers?
To enable optimized procurement decisions for each aspect, it is beneficial to use machine learning to create an algorithm-based approach. This enables organizations to implement dynamic procurement strategies that fully consider market movements. Ultimately, digital tools can boost transparency and provide optimal support for decision making in all four aspects of our approach.
"Especially in times of rapid change and market volatility, it’s imperative for companies to utilize Big Data analytics as their 'compass' in raw material procurement. This is the only way they can navigate the storms of price risk and maintain their profit margins."
How our CostIQ approach can help to reduce commodity purchase prices
With the CostIQ, an AI-powered approach, we can help commodity purchasers consolidate internal and external data on prices, demand, and potential price drivers such as macroeconomic developments or the latest weather patterns. This information can then be used to effectively benchmark the purchasing performance of companies in test environments compared to various procurement strategies. Through this approach, we can offer strategic advice to our clients at the next level by leveraging AI in internal proof-of-concept projects.
With this back-testing approach, CostIQ can identify valid opportunities for cost and risk optimization. It also provides specific purchasing decision-support in order to unlock opportunities to reduce costs and risks. CostIQ helps to
- identify data-driven target prices and trigger price re-negotiations with suppliers
- derive purchase signals on optimal purchase timing and quantity;
- and provide suggestions for optimal index formulas in contractual purchase agreements.
We have carried out in-depth investigations across various commodity classes, including industrial metals such as aluminum, copper and nickel; energies such as natural gas and crude oil; chemicals like methanol; plastics such as polyethylene; and agricultural products like corn and soybean. Ultimately, our research shows that a structured, data-driven and AI-based approach can help companies to reduce commodity purchase prices by an average of 3-5% with minimized price risk.
This article was coauthored by Christian Mandl .
We would like to express our sincere appreciation for his valuable contribution.
Register now to receive regular insights into our Operations topics.